Jules Françoise
I am a CNRS Researcher in Human-Computer Interaction at LISN (CNRS, Université Paris-Saclay) within the AMI Team (Architectures and Models for Interaction). My research focuses on movement-based interaction, interactive machine learning and machine teaching, in the context of creative applications.
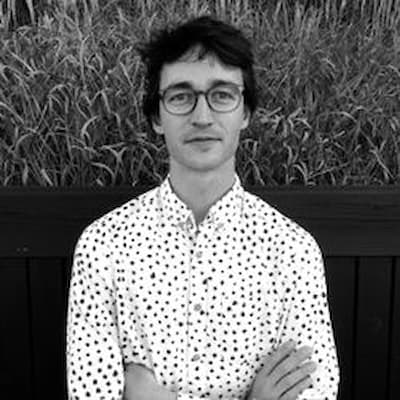